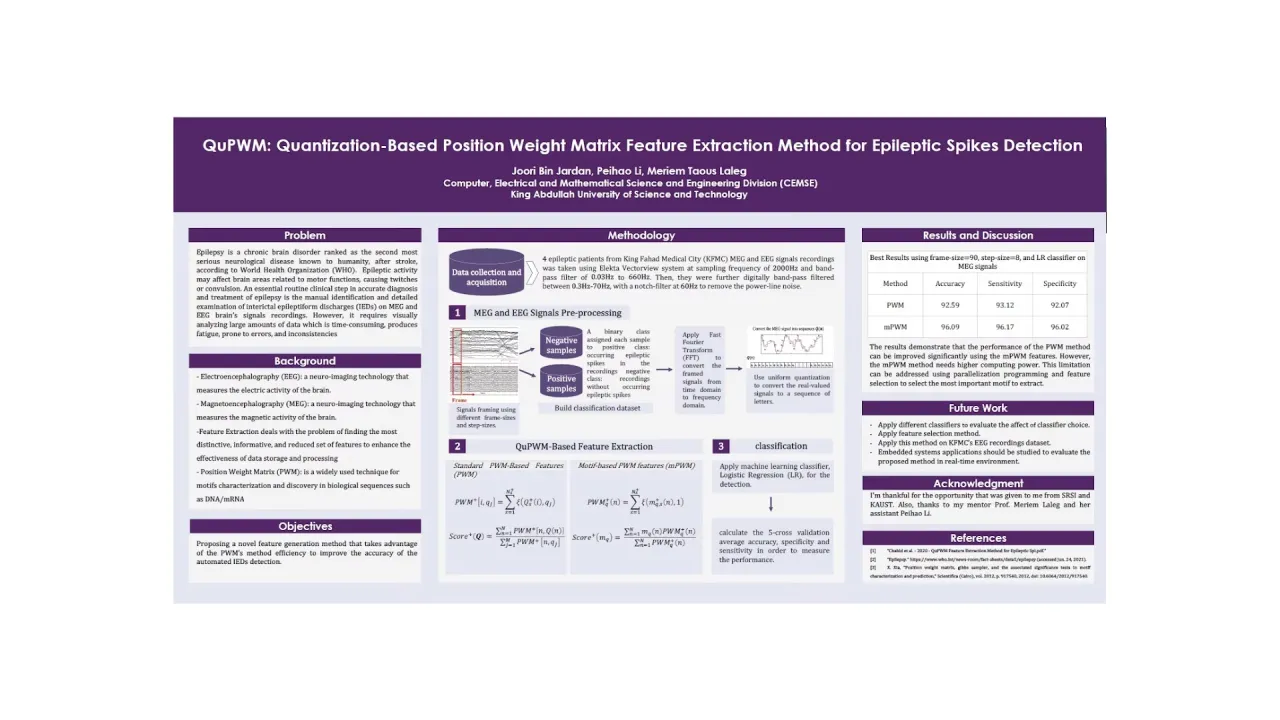
Joori Bin Jardan: QuPWM: Quantization-Based Position Weight Matrix Feature Extraction Method for Epileptic Spikes Detection
- Joori Bin Jardan, SRSI, CEMSE, KAUST, KSA
KAUST Library
Overview
QuPWM: Quantization-Based Position Weight Matrix Feature Extraction Method for Epileptic Spikes Detection
Abstract
Globally, epilepsy is one of the major neurological disorders. According to World Health Organization, it is estimated that approximately 70% of people who suffer epilepsy could live seizure-free life if it was diagnosed and treated properly. After an epileptic seizure, an abnormal neuronal discharge that originates from one or more cranial lobes happens in the brain known as inter-ictal spiking. Often, this abnormal activity interferes with the affected lobe’s normal activity while traveling among different lobes heralding the onset of electrographic seizure. Nowadays, the detection of such irregular neural activity is done using electroencephalography (EEG) and magnetoencephalography (MEG) manual records reading, which is time-consuming and highly prone to errors causing delayed and inappropriate intervention. Therefore, this project aims to integrate the current detection method with an improved machine learning approach that uses Quantization and Position Weight Matrix (QuPWM) to provide an autonomous, time-effective method of epileptic spikes classification from both EEG and MEG signals. Our method, QuPWM, generates useful clinical features from the framed raw signals by using the position weight matrix combined with uniform quantization, after converting them into frequency domain through a Fast Fourier Transform (FFT). Then, feeding these clinical features to the Logistic Regression (LR) classifier for inter-ictal spikes detection. This evaluation was able to achieve a maximum accuracy of 96.09%, using the motif-based PWM (mPWM) features method and the 5-folds cross-validation (CV) technique that is applied on 4 patients from the dataset with a sliding frame of size 95 sample- points and a step size of 8 sample-points. In conclusion, we were able to incorporate machine learning with EEG and MEG recordings to facilitate and provide more accurate diagnoses and treatment of epilepsy, enhancing epileptic patients’ life thereby.
Brief Biography
Joori Bin Jardan is a senior high school Saudi gifted student that studies in the 30th Secondary School in Dammam and has been to several STEM-related enrichment programs. She has been awarded for the top scientific paper in SRSI’21. Joori is aiming to major in computer engineering and become a researcher in the future. Her ultimate goal is to become a successful leader in science and technology.